Multimodal AI: The Future of Intelligent Interaction
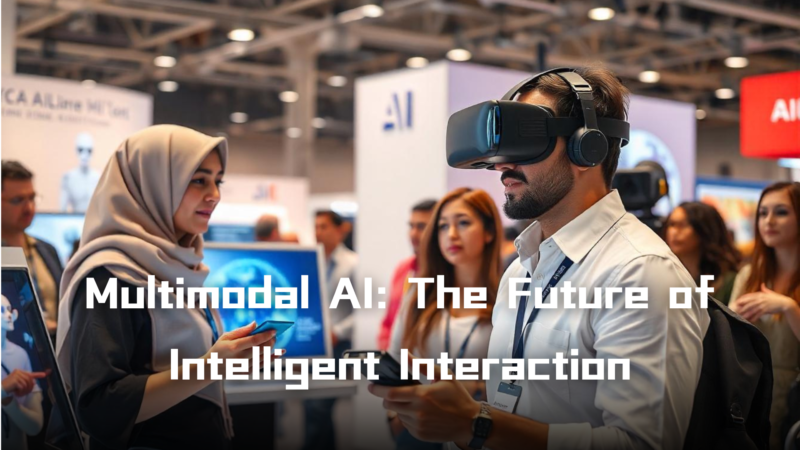
Multimodal AI: The Future of Intelligent Interaction
One of the most intriguing developments of recent years has been the advent of multimodal AI. This cutting-edge technology enables AI systems to process multiple modalities such as text, images, audio, and video all at once. Integrating this information drawn from other sources enables it to provide richer, more nuanced insights and can be very beneficial for augmenting user interactions across various applications. In this blog, we’ll discuss what multimodal AI is, its applications, and challenges.
What is Multimodal AI?
Multimodal AI refers to artificial intelligence systems capable of interpreting and integrating data from various forms of input. Unlike traditional AI models that specialize in a single type of data, like in the case of text or images, multimodal AI can analyze and generate responses based on a combination of inputs. An example of that would be the ability for the system to analyze a video, extract audio, comprehend the visual scene, and process any accompanying textual information.
This extends the ability of AI toward mimicking the human-like understanding, where people naturally integrate information from diverse sources to make opinions or decisions. The most recent terminology includes prototypes where, in a remarkable demonstration of the technology’s potential, Meta’s ImageBind and OpenAI’s DALL-E 3 are integrating various types of data to become more efficient and/completely versatile.(Exploding Topics).
Applications of Multimodal AI
Applications of multimodal AI cover an expanse. Here are key domains where it exerts its influence:
- Healthcare: Multimodal AI faces solutions for medical diagnosis through analyzing imaging data along with electronic health records along with clinical notes. With the use of this technology, diagnostic predictions and tests will be more accurate than classical ones. For example, visual data (obtained from scans) when integrated with textual data (from patient histories) can yield improved treatment plans.
- Autonomous Vehicle: The development of self-driving vehicles greatly depends on multimodal AI systems. These vehicles can make real-time decisions in complicated driving scenarios by integrating data from cameras (spatial), LIDAR (environmental), and other sensors .
- Creative Content Generation: Programs such as DALL-E and Google’s Gemini allow the generation of images on the basis of textual descriptions. As these models begin to mature, they are in fact beginning to add other modalities to their repertoire, for instance, to generate short videos or music clips based on user prompts.
- Augmented Search: Multimodal AI has elevated search engines to a level where search results now offer candidates that are combination results of images, videos, and text, all tailored to user queries. This gives users the opportunity to look for information in a much more effective manner .
- Natural Language Processing: In customer service, multimodal AI can analyze chat transcripts, voice calls, and sentiment from customer interactions in order to provide a better support and personalize that user’s experience .
Challenges of Multimodal AI
Multimodal AI, with its capabilities, has a set of challenges that must be overcome for the final sake of successful applicability and implementation through research and development:
- Data Integration: The Coherent Solution The technical integration problem is the variance in data format and the requisite context which complicates the process for making correct interpretations.
- Computational Complexity: The training and deployment of such multimodal AI systems require tremendous computing power. This would make it more challenging for any organization to fund as well as deploy more quickly, particularly those with limited resources .
- Bias and Fairness: As with any other type of AI technology, multimodal systems inherit biases in their training data. The acceptable ethical usage of these systems will come to be a critical issue when they are fair and unbiased across different modes.
- Integration of multiple types of data raises concerns regarding users’ privacy and security of their data. There must be standards and frameworks for ethical use.
The Future of Multimodal AI
The future of multimodal AI looks promising. As technology advances and researchers overcome existing challenges, we can expect to see even more sophisticated applications across various fields. The ongoing development of models that can effectively process and integrate multimodal data will likely enhance user experiences, improve decision-making processes, and drive innovation in countless sectors.
In conclusion, multimodal AI represents a significant leap forward in the field of artificial intelligence. By enabling machines to understand and respond to a diverse range of inputs, this technology holds the potential to revolutionize industries and improve our everyday lives. As we navigate this exciting frontier, the focus on ethical practices, privacy, and bias will be vital to harnessing the full benefits of multimodal AI.
Important Link
- How did Kris Kristofferson die
- How to sign in bank of America online
- Millie Bobby Brown and Jake Bongiovi: A Love Story for the Ages
- India Women vs South Africa Women Cricket Match 2024
- Is Iran attacking Israel
- What happened to John Amos
- How many leagues are there in sports
- What are sports leagues
- How many times India beat Bangladesh?
Disclaimer: chronobazaar.com is created only for the purpose of education and knowledge. For any queries, disclaimer is requested to kindly contact us. We assure you we will do our best. We do not support piracy. If in any way it violates the law or there is any problem, please mail us on chronobazaar2.0@gmail.com